Generative AI in Science: Real-World Applications and Simple Explanations
How Generative AI is Transforming Scientific Research and Helping in the Lab
AMP Version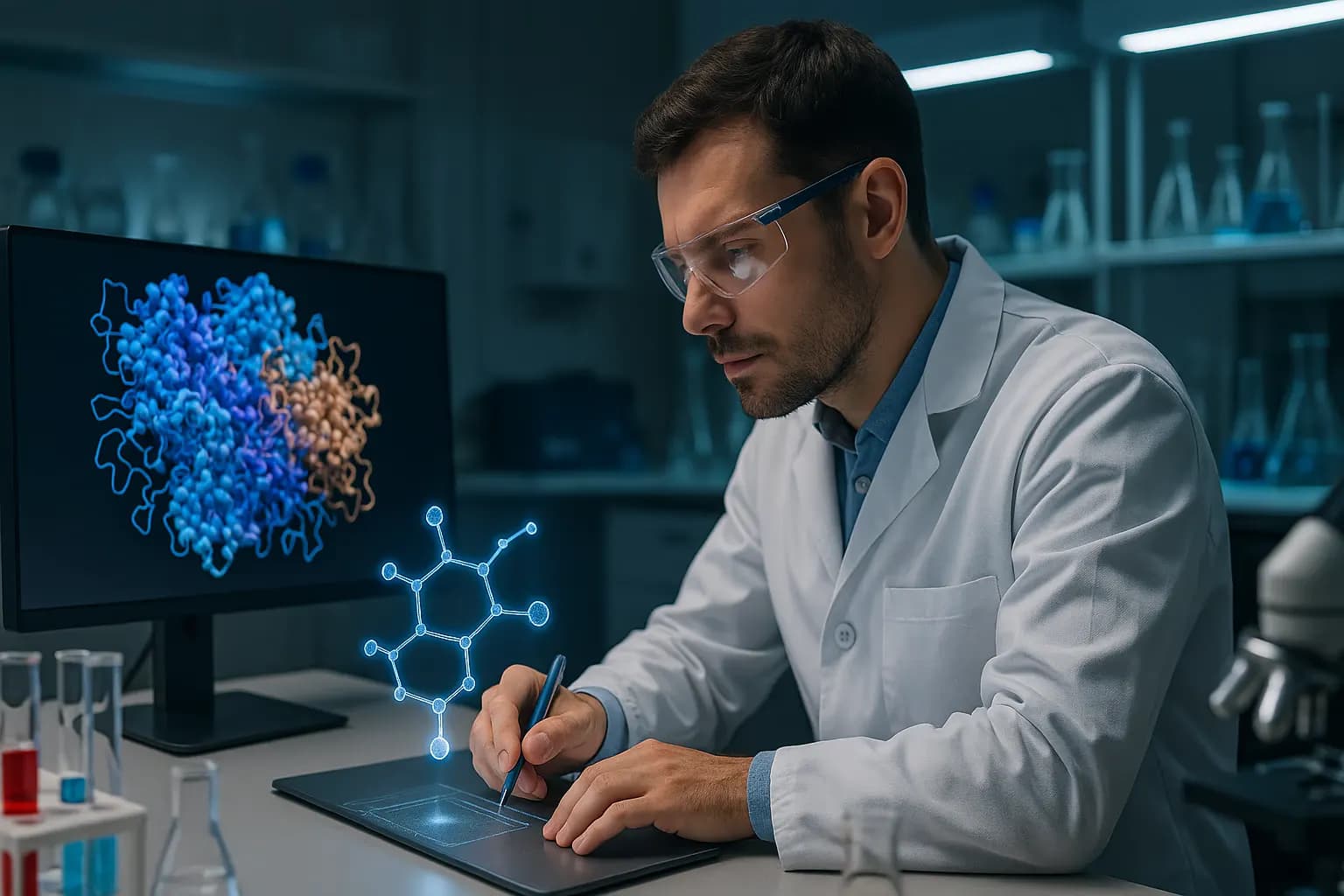
Introduction
Generative artificial intelligence (AI) is a rapidly evolving system that can create entirely new content, from text and images to complex scientific data and hypotheses. In this article, we focus on real-world scientific applications of generative AI, avoiding unnecessary technical details. Our goal is to explain in simple terms how these innovative tools are already helping scientists accelerate research and make discoveries today, and what trends will shape their role in science throughout 2025.
1. Biology and Structural Bioinformatics: Decoding the Language of Life
Understanding the 3D structure of proteins is critical for biology and medicine, where generative AI has made a real breakthrough.
* AlphaFold2 (DeepMind) and RoseTTAFold (University of Washington and Meta AI) predict a protein’s 3D model from its amino acid sequence with astonishing accuracy. This saves months or even years of lab work.
Example: Enzyme scientists visualize the active site, understand how it works, and develop inhibitors that can precisely target protein regions.
2. Molecule Design and Drug Development: From Idea to Candidate in Days
Creating new drugs is a long and expensive process. Generative AI offers a revolutionary approach to speeding it up.
* Insilico Medicine (Chemistry42, PandaOmics) generates molecules with specified properties (high bioavailability, low toxicity) and evaluates their potential therapeutic efficacy.
* NVIDIA BioNeMo is a cloud platform that suggests compound options and predicts their properties and toxicity.
Example: instead of months of manual selection, researchers receive hundreds of promising candidate molecules in a few days, which significantly reduces the early stages of drug development.
3. Automated Analysis of Scientific Literature: Navigating the Ocean of Knowledge
The volume of scientific information is growing exponentially, making it increasingly difficult for scientists to keep track of relevant research.
* Elicit, SciSummary, Consensus AI and other services sift through thousands of publications, extract key findings, and generate structured reports or summaries.
Example: a student or researcher starting a new topic (e.g., “RSV vaccines”) can get a list of the most important articles with key points and answers to specific questions in minutes.
4. Help with writing scientific texts: AI as a scientific assistant
Generative language models are becoming indispensable assistants in preparing scientific publications:
* ChatGPT (OpenAI) and Claude (Anthropic) create draft introductions, help reformulate complex phrases for clarity, improve style, and check grammar.
Example: a researcher enters an article plan and key results, and the model generates a coherent draft text that requires further verification and revision.
5. Tips for an effective start and work in 2025
- Define the task and choose a tool: AlphaFold2 or RoseTTAFold for structures, Elicit for reviews, ChatGPT/Claude for text.
- Formulate queries clearly and specifically: "Predict the 3D structure of a protein from the XYZ sequence" or "Prepare a brief review of studies on the impact of microplastics on marine ecosystems over the past 3 years."
- Always check the results: check the correctness of the numbers, scientific terms, and conclusions with the original sources.
- Indicate the use of AI: add a note in the "Methods" or "Acknowledgments" section about the use of generative AI.
Prospects and challenges for 2025 and beyond
* More complex and accurate models: AI will be able to solve even more complex scientific problems.
* Multimodal systems: working with text, images and structural data simultaneously.
* Hypothesis generation: AI will suggest new scientific ideas for testing.
Challenges:
- Quality and availability of training data.
- Interpretability ("black box") of models.
- Ethical issues: falsification, authorship and transparency.
- Preservation of critical thinking and the leading role of humans.
Conclusion
Generative AI is no longer just a futuristic concept, but a real working tool that radically changes approaches to scientific research. It helps to model complex systems, accelerates the development of new materials and drugs, systematizes huge flows of information and assists in the preparation of scientific publications. These technologies make science faster, more efficient and accessible.
2025 promises to be a period of even more active implementation and development of these tools. Start exploring their capabilities today: many services offer free or trial versions. Set your first scientific task for generative AI - and you will be surprised by the results obtained and the prospects that open up! The main thing is to approach this process consciously and always remember the role of the scientist as the main conductor of the research process.
Now you know how Generative AI helps scientists reach new heights.